Getting to the Root of Dental Defect Detection With Synthetic Data and Machine Learning
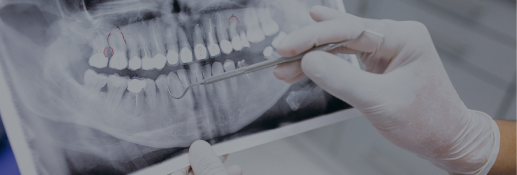
Challenge
Dental X-rays are a snapshot of patient oral health, but don't always give doctors and insurers the clarity needed to treat patients. In conjunction with a Seattle-based dentist to build, we developed a proof of concept using visual synthetic data and machine learning to bring about more accuracy.
Outcome
The combination of visual synthetic data and machine learning yielded a surplus of data points that better enable insurers and doctors to identify dental defects. These insights can lead to more proactive treatment plans and, potentially, better overall patient and provider experiences.
Dental radiograph examination can be a prolonged and inexact science for providers. To make it a faster, more efficient process, our team developed a proof of concept using machine learning and synthetic data principles.
Machine learning (ML) and synthetic data are primarily associated with automation and other next-generation bespoke solutions, but there’s value in integrating them into other industries and processes. To illustrate the breadth of synthetic and ML use cases, our team developed a proof of concept to demonstrate how these solutions could help insurers and dental providers analyse radiographs more effectively and efficiently.
Providing clarity into ongoing issues
For almost 130 years, X-ray technology has been informing healthcare diagnostics and decision-making. But it’s not without struggle for insurers that collaborate with dental health providers. Those issues typically boil down to:
- Radiograph inconsistency: The quality and consistency of dental radiographs vary, complicating the claims approval process.
- Significant time investments: Due to the high volume of imagery, assessing radiographs and processing claims becomes time intensive.
- Required expertise: The necessity for expert evaluations of each claim introduces potential bottlenecks, from processing delays to scaling challenges.
Understanding the universality of these issues, we worked with Dr. Paul R. Amato, DDS, FAGD to conceptualise a radiograph assessment algorithm. Dr. Amato collaborated with us to distil his team’s knowledge down into repeatable, scalable steps. With his domain expertise, our goal was to empower synthetic-data-driven ML to act and streamline the approval process for patient diagnosis and treatment plans.
Solving operational logjams
In this proof of concept, we focused on bitewing (both levels of teeth on one side of the mouth) and periapical (a root-to-crown image) radiographs. We crafted synthetic representations of these views by:
3D replications
Using a 3D scan of a human skull, we generated variations of human teeth to simulate the maturation process from child to adulthood. To build internal structures of the jawbone and teeth represented in radiographs, we used volumetric rendering with low- and high-frequency noise patterns to replicate the biological structures of the mouth as they appear in radiographs.Anatomical accuracy
We developed systems to represent the enamel, dentin, and pulp layers of the teeth, recreating their actual densities to replicate how X-rays are absorbed during a radiograph. We then introduced defects (i.e., lost density for cavities, added density for fillings) and used scattering methods to accurately distribute them within the tooth. Each tooth and defect type received a label corresponding to its category that is used during the machine learning training process to inform the network of the information we wanted it to learn.Emulating radiographs
We positioned 14 cameras in specific parts of the mouth to produce data that is accurate to how radiographs are collected in a clinical setting. Then, we added positional variation on top to represent variations that might happen during real-world captures. We also introduced non-defect variables to represent the variance in the human population, not overfit to a particular demographic and allow for more generalised detection options.
Accelerating solutions
In a matter of two weeks, our model improved and was able to accurately identify individual teeth and specific conditions. In this process we obtained insight into the benefits of machine learning and synthetic data for in the dental field.
- Enhanced Diagnostic Models: Boost the accuracy and dependability of dental diagnostic models by training them on diverse and lifelike synthetic X-ray datasets.
- Optimized Radiographic Imagery: Fine-tune dental radiographic visuals under varied conditions, ensuring clarity and precision for dental practitioners and insurers.
- Radiograph Privacy and Compliance: Rely on realistic, non-sensitive synthetic X-ray data to maintain patient privacy and align with data protection regulations.
- Unlock Advanced Dental Analysis Features: Detailed annotation capabilities made possible by synthetic data make the development of cutting-edge diagnostic features possible.
- Accelerated Radiographic Innovations: Rapid develop and fine-tuning of datasets and machine learning features eliminates the hurdles of traditional data collection and annotation.
This demonstration provided a glimpse into how ML and synthetic can combine their unique abilities to create innovative solutions in a challenging domain. Furthermore, it acted as a preview of the broad range of opportunities visual synthetic data can provide in the healthcare and insurance industries.
"I am excited for the future of machine learning in the dental world especially when it comes to radiographs. The extra layer of a software program that can assist me in detecting cavities, infections and anything abnormal allows my patients to receive the most advanced and comprehensive care that is possible.” said Dr. Paul R. Amato, DDS, FAGD.
For a more in-depth discussion about our work with Amato Dental, watch our presentation on it from SIGGRAPH2023 here.
Explore the industry
Technology
Give your technology the push it needs to reach the next level of efficiency – and break new ground!
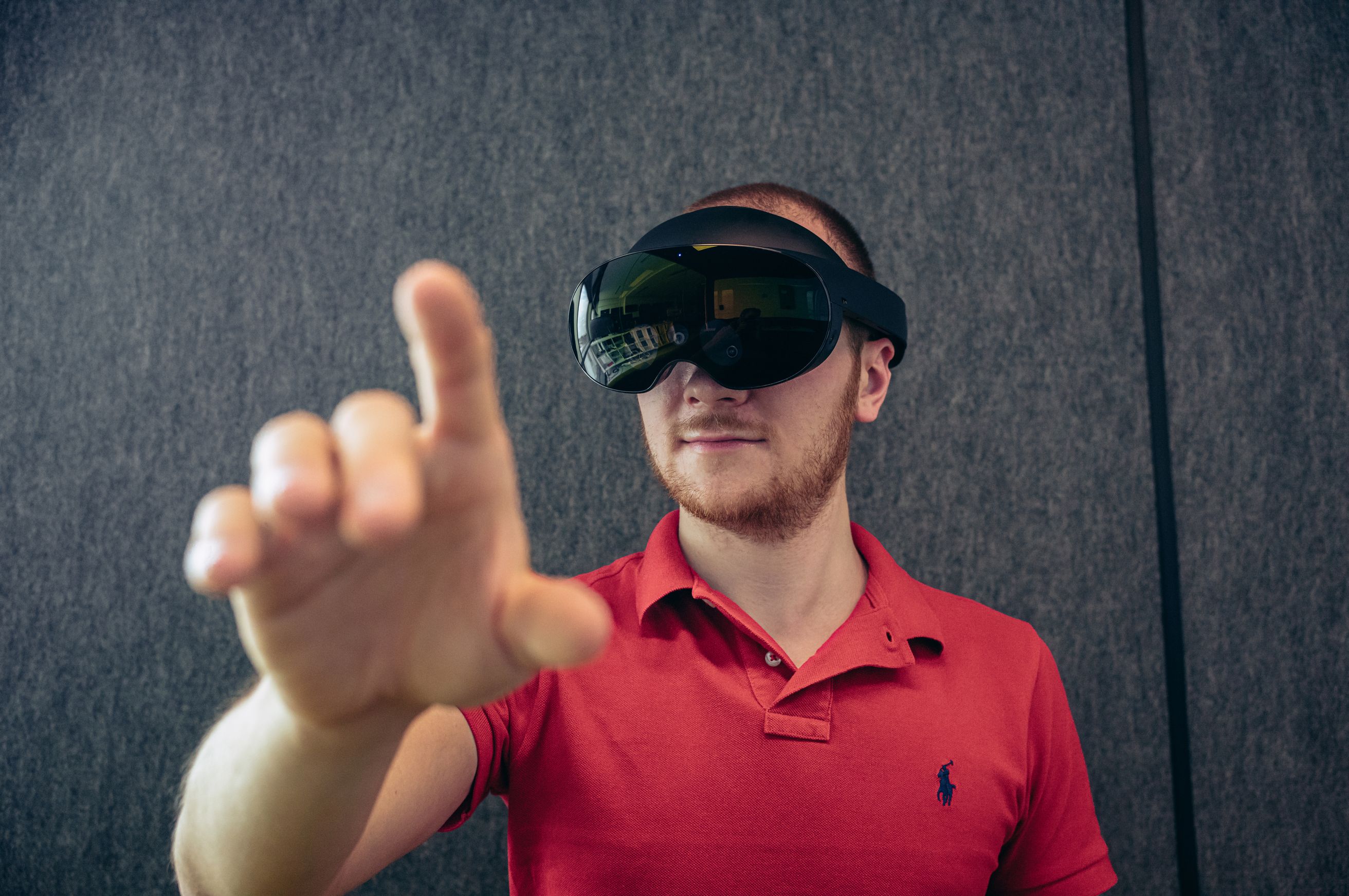